As a social science researcher studying trust and safety on online platforms, it is critical to think through potential risks and negative impacts associated with the use of generative AI technologies. To be sure, its potential is incredible – and will benefit societal members in almost limitless ways. In this environment of giddy euphoria about its use, though, it seems wise to consider how it might be used to cause harm – and what we need to be doing to reduce potential fallout from its misuse.
What is Generative AI?
Generative AI is a type of technology that uses computer algorithms to “generate” new and original things, like images, videos, music, or even text. Generative AI is trained on large amounts of content and analyzes it to understand patterns, styles, relationships, and unique characteristics within the data. For instance, it might examine pictures of Marvel Cinematic Universe protagonists, and learn what a typical character looks and acts like. It might analyze a selection of popular songs from the 2000s and learn what they have in common during that era.
Once it has learned from the data, it can start creating new things on its own. If it’s trained on images of planetary landscapes, it can generate new images of planetary landscapes that look realistic and otherworldly, even though it has never seen those exact images before. The technology uses its continuously evolving understanding of patterns and styles to make something new that fits in with what it has learned. Generative AI is exciting because it can create things that seem like they were made by you or me. It can come up with content that is new and different than what we are used to, and produce interesting and fresh variations upon what we might expect.
ChatGPT is probably the most famous text-based generative AI system to date, while Microsoft CoPilot, Google Gemini, and even Snap’s My AI are all gaining traction. MidJourney, Dall-E 3, and Craiyon have received attention for producing amazing images, Stable Diffusion and Runway for producing videos, and Murf and Lovo for producing voice. These are constantly being updated, and other tools based on ever-improving models continue to come down the pike every single week. It is easy to get caught up in these shiny new objects without taking the time to learn and reflect upon how to use the tools in ethical and responsible ways. But failing to appreciate the potential risks not only sets us up to be blindsided, but also undermines the promise of generative AI to meaningfully improve our work and play. Will it be used for good – to create new forms of art, to advocate for social justice issues, to achieve measurable progress? Or will it be used for ill – to harass, deceive, or otherwise victimize others?
Below I’ve sectioned out different types of harm that seem primed for perpetration through the use of Generative AI technologies (and overlap exists in terms of how each harm is carried out). In no particular order, these include: harassment and cyberbullying; hate speech; deepfakes; catfishing; sextortion, doxing and privacy violations; dogpiling and report brigading; and identity theft/fraud. This is not an exhaustive listing; we know, for example, that disinformation and fake news is another problem that will naturally grow with the use of this technology. Our Center focuses on direct forms of interpersonal victimization and as such we will stay in that lane in the discussion below.
Harassment and Cyberbullying
Generative AI allows for both the automatic creation of harassing or threatening messages, emails, posts, or comments on a wide variety of platforms and interfaces, and its rapid dissemination. Its impact historically may have been limited since it takes at least some time, creativity, and effort to do this manually, with one attack after another occurring incrementally. That is no longer a limitation, since the entire process can be automated. Depending on the severity, these generated messages can then lead to significant harm to those targeted, who are left with little to no recourse to stem the voluminous tide of abuse, nor identify the person(s) behind it. Reports indicate that harassment via automated troll bots is a significant problem, and it seems only a matter of time that real-time, autonomous conversational agents take over as a primary vehicle for, or driver of, harassment. At best, it is incredibly annoying and at worst, it can overwhelm victims, greatly amplify the impact of the harassment and cyberbullying, create a hostile online environment, and lead to substantial psychological and emotional harm.
AI-generated harassment and cyberbullying is incredibly annoying and at worst, it can overwhelm victims, greatly amplify the impact of the harassment and cyberbullying, create a hostile online environment, and lead to substantial psychological and emotional harm.
Furthermore, generative AI algorithms can take online attacks to a higher level by learning from granular-level data available about a person. It can analyze a target’s social media posts, online activities, or personal information to generate highly specific and threatening messages or content. It can create output that references specific locations, recent events, or private details about the target’s life after learning as much as possible about them, making the harassment much more personal and intimidating. Think about all that you (or your teenager) share(s) online, and how it might take a malicious individual days, or even weeks, to sort through all of the captions, comments, pictures, videos, and livestreams to build a complete dossier about them. Now, an aggressor can use generative AI to do it in minutes (or less).
One might hope that content moderation teams at each online platform have created technology to mitigate the reach and impact of these harms. However, it is arguable that they are often behind the proverbial 8-ball and regularly playing catch up to the creative approaches of wrongdoers. Generative AI can be employed to produce content that successfully evades or bypasses automated content moderation systems given what the model learns about how an online platform operates. A simplistic example would be autonomously creating content with periods or numbers in the place of certain letters that make up certain sexually-offensive terms. A more complex example would be creating subtle variations or modifications in offensive pictures, threatening videos, or other prohibited content to circumvent detection, deletion, or deprioritization.
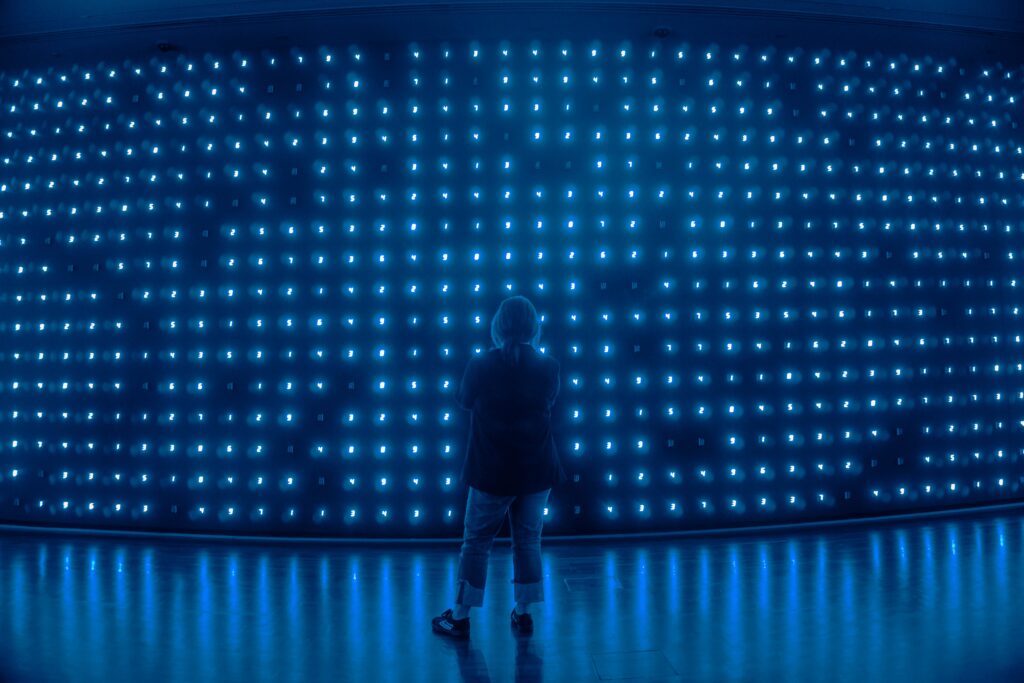
Hate Speech
On March 23, 2016, Microsoft released a chatbot named Tay, a machine learning project that was described as “an experiment in conversational understanding.” The more that people chatted with Tay, the smarter it became as it learned words, language, and phrases and then parroted them back. Only 24 hours later, however, it had become a vehicle for hate and harassment because a swath of individuals began tweeting at it with racist and misogynistic statements targeting Jews, women, and others in horrific ways. Dutifully, Tay then learned these expressions and repeated or iterated upon them to other users. Despite Microsoft’s efforts to build Tay using “relevant public data” that had been filtered and vetted appropriately, at the time they clearly hadn’t thought through some of the possibilities when it operated in real time. Within another day or so, Tay was shut down for good.
We have come a long way since 2016, and generative AI models have moved light years ahead in helpfully and accurately responding to our inputs and prompts. When used maliciously, though, Generative AI can create and propagate large volumes of hate speech in ways that spread like wildfire. This can manifest in general ways with no specific targeted individual in mind, as well as against certain groups based on their unique identities (e.g., race, gender, sexual orientation, or religion), beliefs, or affiliations, the effects of which can be intimidating and downright scary. It can also have an even greater chilling effect if set up to target particular individuals who are trying to speak out against hate speech and marginalization. Not only can AI help it spread, but also be amplified and rendered way more visible as algorithms are built to automatically like and share the content – and increasing the following of the original poster – thereby gaming the system to increase virality. Generative AI can even create fake news or otherwise misleading content that further marginalizes and victimizes certain communities.
Not only can AI help spread hate speech, but also be amplified and rendered way more visible as algorithms are built to automatically like and share the content – and increasing the following of the original poster – thereby gaming the system to increase virality.
Another major concern has to do with the quality of data on which generative AI models are trained. If the corpus of data analyzed includes racist, sexist, homophobic, misogynistic, or otherwise harmful language or imagery, reflects societal biases and stereotypes, and lacks diversity and equal representation among the people who originally generated it, discrimination might be resident (and prominent!) in the new content it creates and shares. These biases due to the training data or the model design can manifest in various ways: the preferential generation of content from certain demographics and not others; the reinforcement of stereotypes by building and propagating harmful narratives; and the production of other outputs that characterize or label others in very offensive ways.
Deepfakes
In the summer of 2020, a unique case of harassment came to light as a 50-year-old woman used technology to target some of her daughter’s peers in their Pennsylvania community. The most interesting twist was not the vast age difference between the aggressor and the targets, but that fact that software was used to algorithmically alter original images found online to make it seem like the other girls—who belonged to a cheerleading club that her daughter previously attended—were nude, engaged in underage drinking, and/or using vaping products (prohibited by the community gymnasium). These “deepfakes” were then spread via harassing texts from anonymous phone numbers unrecognizable to the girls. Interestingly, this case was one of the first we’ve used in our university classes as to how generative AI can be employed as a vector for harm and harassment.
The term deepfake (deep learning + fake) itself may have originated back in 2017 when some online users began sharing their own creations of fake celebrity porn with each other. As explained earlier, models are created by using computing power to analyze significant amounts of image-based content (e.g., hours of video of a person, thousands of pictures of a person—with specific attention to key facial features and body language/position). Then, what is learned is applied to the images/frames one might want to manipulate or create (e.g., superimposing your face upon a nude body, placing your entire self in a scene that calls into question your reputation, creates content that makes it seem like you are saying something you would never say). Additional techniques such as adding artifacts (like glitching/jittering that appears normal or incidental) or using masking/editing to improve realism are also employed, and the resultant products are (unsurprisingly, given how fast these technologies have developed) convincing.
Recently, a woman on TikTok (link defunct) shared a moving video explaining how she was victimized by a man who took her completely-clothed images and used AI software to create nudes of her. She even provides details to her audience of followers to prove that the images are fake: “underneath the left hand there is a chunk of black where they were unable to completely erase my top” and “there are lines where my tattoos dont line up and folds on my body that arent there. My chest is much smaller than in the edited photos. I don’t have any tattoos on my lower uterus area.”
But recognizing and even verbalizing that they are not real does not attenuate the harm that deepfakes can cause. There are countless other stories detailing similar experiences involving generated pornography. And we’ve already detailed how quickly and efficiently this type of content can be broadcasted far and wide, thereby causing significant emotional and psychological harm, damaged personal relationships, and long-lasting consequences to the victim.
(As a quick aside, research is being done on AI generated child sexual abuse videos which are trafficked in the worst corners of the Internet. Additionally, “deepnude” apps can generate explicit content from images of clothed individuals, and if minors are involved this can significantly contribute to the global problem of child pornography and child sexual exploitation.)
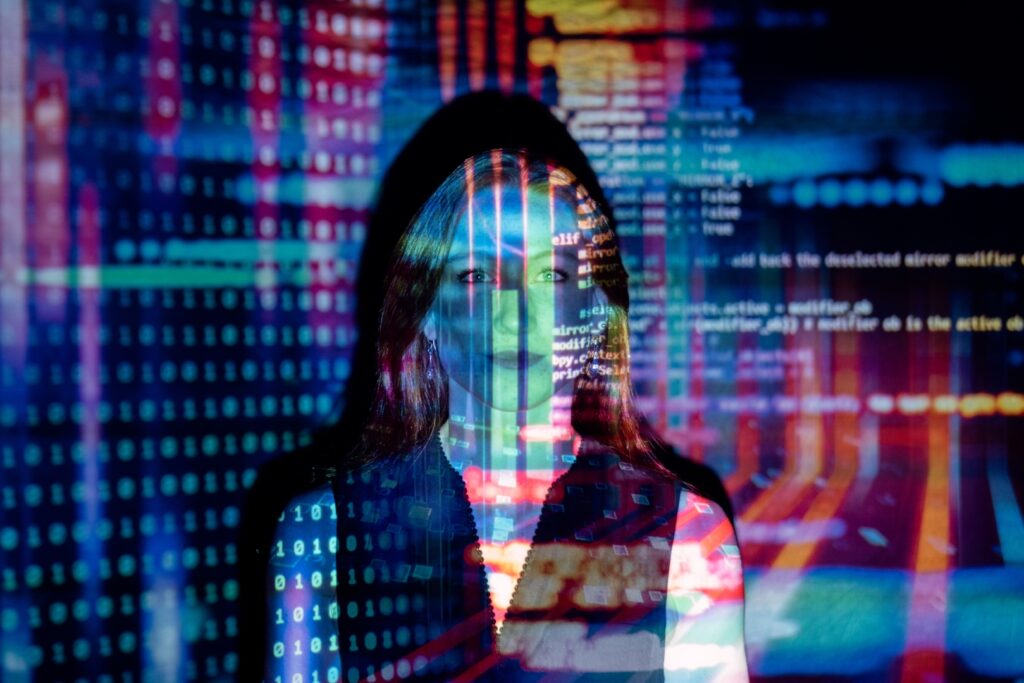
Catfishing
Catfishing involves the creation of fake online identities to trick others for fun, romantic attention and affection, monetary gain, or another self-serving reason. By now it should be easy to imagine how AI can generate text, images, and video given the descriptions and examples above. As such, it should not be a stretch to envision how it can be instructed to build very realistic social media profiles with believable biographical sketches and other details to convince users of its legitimacy.
By way of example, a couple of computer scientists in early 2023 created an AI avatar of a 19-year-old female named “Claudia” using Stable Diffusion just to see if they could fool some unsuspecting users on Reddit. Soon after, they were found out, but not before earning $100 by catfishing others into paying for additional AI-generated nudes of Claudia.
Generative AI can be used to build conversational agents or chatbots that allow AI avatars to appear real by simulating dialogue and even building emotional connections with their targets. By flirting and feigning romantic interest, these chatbots can lead individuals to believe they are interacting with a genuine person.
The creation of a fake but convincing profile, though, is helpful only to a certain extent, and then requires charisma, deception, and effort on the part of the catfisher. Now, generative AI can be used to build conversational agents or chatbots that allow AI avatars to appear real by simulating dialogue and even building emotional connections with their targets. By flirting and feigning romantic interest, these chatbots can lead individuals to believe they are interacting with a genuine person. Indeed, AI can automatically generate continual messages or content that align with the target’s interests, values, or preferences after analyzing that target’s online presence, behaviors, and statements, rendering the communication very personalized and seemingly trustworthy. The fact that all of this can happen in real time to deepen intimacy and solidify trust can truly build a case that this created persona is actually a real person, and that all they have expressed is heartfelt and legitimate. In this setting, is it easy to see how our next form of harm might ensue.
Sextortion
Generative AI can contribute to sextortion, a form of online blackmail where individuals are coerced or manipulated into providing explicit images or engaging in sexual activities under the threat of having their intimate content exposed or shared without consent. We’ve already discussed deepfakes above, and it is not a stretch to envision how highly realistic and deceptive content that appears to depict a target engaging in sexual activities can be used to blackmail or extort unless that target complies with the aggressor’s demands. As mentioned, this can be neatly coupled with the use of AI chatbots to initiate and sustain interactions with targets. Aggressors can program these conversational agents to engage in dialogue that gradually escalates in a sexual nature, encouraging and inducing targets to share (and/or reciprocate) nudes or other explicit content. Once the content is obtained, it can be weaponized to facilitate sextortion.
Doxing
Given the web crawling, scraping, and learning capabilities of generative AI technologies, one might easily envision how it can be employed to compile and publicly disseminate personal information about others without permission. Known as doxing, this specific form of harassment typically is done by aggregating and analyzing data obtained by deeply examining websites, public databases, social media posts, and other repositories of content. What sort of information are we typically talking about in doxing cases? Most often, it is personal contact information such as a phone number, email, or home address, but could also be related to something that a person doesn’t want disseminated, like sexual orientation, sexual experiences, physical or medical issues, family problems, or anything else that someone is not (yet) comfortable sharing. Apart from automating these actions, AI algorithms also can help generate and share content that triggers online shaming campaigns (enlisting a critical mass of online users to “cancel” someone else), encourages swatting (e.g., where the police are tricked into sending an armed response team to someone’s address through the false reporting of a bomb threat or hostage situation), sends unwanted deliveries to an address, or stalks and threatens individuals.
AI algorithms also can help generate and share content that triggers online shaming campaigns (enlisting a critical mass of online users to “cancel” someone else), encourages swatting (e.g., where the police are tricked into sending an armed response team to someone’s address through the false reporting of a bomb threat or hostage situation), sends unwanted deliveries to an address, or stalks and threatens individuals.
A recent example that made the news involved harassers posting AI-generated sound clips of various voice actors on social media in an attempt to incite violence against them. For example, one Twitter post shared the fake voice of an actor which read out their own home address and then stated “I live in the [homophobic slur] city that is Los Angeles. Yes, that does also mean I live in California, the most [racist slur] state in the USA. Personally speaking, killing [racist slur] and [sexually abusive act] children is completely fine.” To be sure, any individual whose voice has been shared online runs the risk of similar victimization because of the ease of access to AI-based voice synthetization software online, and the plethora of public venues in which this information can be shared with relative impunity. Privacy is first invaded when the personal information is furtively acquired without authorization, and then violence is incited if it is then shared with an intent to intimidate, silence, scare, or otherwise cause harm.
As a final point here, the way in which generative AI models might facilitate doxing is congruent with how cyberstalking is aided by the technology. The first step would be to analyze public and semi-private information including social media posts, browsing history, geolocation data, and message logs from various online interactions, detailed profiles of individuals can be built. The next step would be to specifically generate content that demonstrates an invasive knowledge of the target’s activities and movements, creating a sense of constant surveillance and perpetuating an environment of fear.
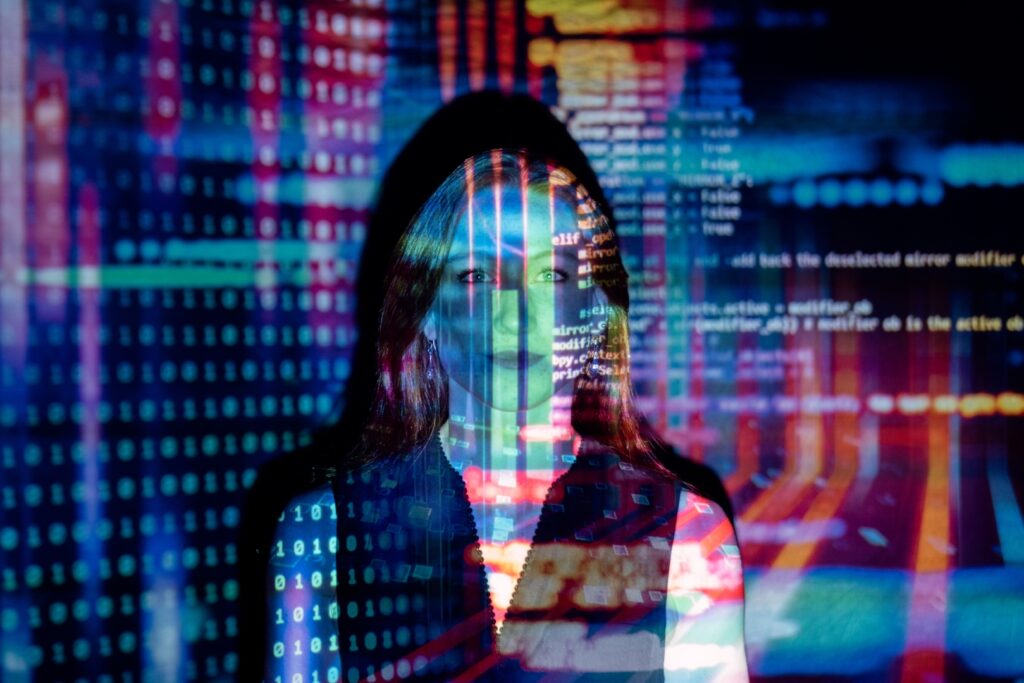
Dogpiling and Report Brigading
While I haven’t yet seen a news story covering the topic, it is reasonable to assume that dogpiling and report brigading will be expedited through generative AI. If you’re not familiar, dogpiling is when users in a chat, comment thread, or similar online venue “pile on” a target with a high quantity of repeated hateful and harassing remarks. Algorithms can be written to facilitate these harmful behaviors by constantly generating unique chatbots to spew content that denigrates or demeans a user, and in an overwhelming, rapid-fire manner. Report brigading is when an individual (or group of individuals) launch a coordinated attack against someone by formally reporting them for fabricated misdeeds to a platform in an effort to trigger a punitive response (e.g., an account deletion, and IP address or hardware ID ban) (or to just harass them mercilessly). AI can generate these false reports with incredible ease and rapidity and make it seem as if they are coming from different “users.” Through these techniques, it’s very possible the platform may not be able to discern that they are part of an orchestrated act of online violence. Sanctions may be levied unfairly, and users who did nothing wrong could be doubly victimized – first by the targeted abuse and secondly by losing privileges to remain on the platform.
Identity Theft
Four years ago, a UK-based energy firm executive wired over $200,000 to a company supplier after his boss told him to do so urgently via a phone call. It turned out, however, that the company supplier was actually a criminal, and the “boss” was actually an AI-generated voice. Think of how the technology has improved since then, and also think about how a number of companies (like my stock brokerage) use voice ID verification. Termed vishing (voice phishing), this illustrates the possibilities of how generative AI can be marshalled in order to deceive and rip off unsuspecting individuals.
Much like the creation of fake accounts to catfish unsuspecting users, generative AI can construct false identities to commit numerous types of online fraud. Such identities can attempt to social engineer passwords and personal information from others via highly intelligent, crafty, and persuasive conversational elements designed to cajole and deceive. AI profiles or personas might also engage in phishing campaigns to mimic legitimate organizations or individuals, increasing the chances of success in luring victims into divulging confidential information. Relatedly, algorithms can be written to aggregate and analyze large bodies of files that hold medical, financial, academic, or personal information, and then generate counterfeit documents that are almost impossible to distinguish from legitimate papers. Of course, official signatures can also be forged in this manner to facilitate theft or fraud.
Let’s Not Forget About the Positives
At the onset of this piece, I mentioned the vast potential of generative AI to improve our lives. As such, I want to try to end on a bit of an upswing. Generative AI has numerous positive and prosocial applications that should not be neglected or minimized, and I am particularly impressed and inspired by some of the stories I’ve heard involving youth leveraging the technology to foster social good. For instance, a group of high school students created an AI-powered chatbot called “Chloe” to support students dealing with mental health challenges. Chloe provided information on coping strategies, self-care techniques, and suggestions for seeking professional help when needed. As another example, a group of students in Canada developed an app called BlindSight to assist individuals with visual impairments. Specifically, it takes images captured by the camera and uses AI algorithms to analyze them and provide real-time audio descriptions of the surroundings. It can identify objects, colors, people’s expressions, emotions, and even read text from signs or books aloud, while also helping impaired individuals get to where they need to be. Both of these projects highlight the incredible way that generative AI can be creatively harnessed to support, protect, and enhance the lives of others and, as a result, make the world a better place. And these are only two of many, many thousands of innovative use cases that endeavor towards that same end.
Given this, the future is bright when it comes to the application of generative AI, but the concurrent potential for misuse and harm demands vigilance and intentionality across all stakeholders to prevent abuse. Next week, we will examine and parse out the role of both users (you and me!) and online platforms in forestalling victimization. We’ll cover topics such as the education of the userbase, the value of policies, how content moderation should be refined, technological safeguards, and collaborative opportunities between researchers, governmental authorities, and the companies who share a clear responsibility to act proactively, ethically, and quickly to keep harm at bay. More soon!
Suggested citation: Hinduja, S. (2023). Generative AI as a Vector for Harassment and Harm. Cyberbullying Research Center. https://cyberbullying.org/generative-ai-as-a-vector-for-harassment-and-harm
Featured Image: Sanketgraphy
Inline Images: Y K, ThisIsEngineering, and DeepMind